
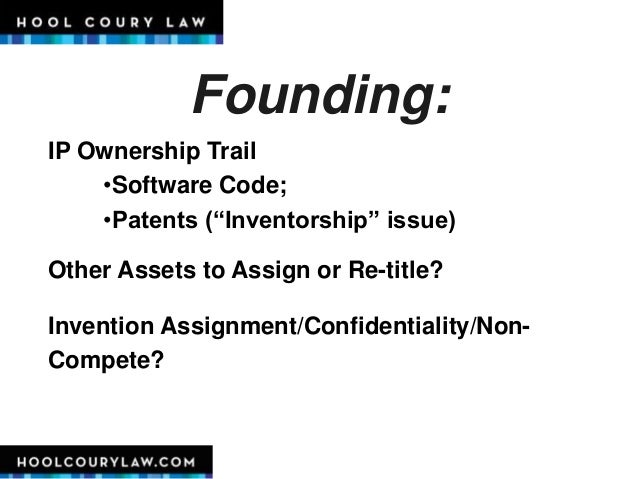
When it comes to launching machine learning-powered products, competition is defined not only by network effects and features, but also by AI factories: Building profitable applications on GPT-3 So, no matter how good an Amazon clone you create, unless you can bring a critical mass of buyers and sellers to your platform, you won’t be able to snatch the competition away from the “everything store” in a profitable and sustainable way. Sellers continue to sell their wares on Amazon because that’s where the buyers are. So why hasn’t any other product dethroned the ecommerce giant? Amazon has built a hefty “moat” around its platform through network effects: Buyers continue to go to Amazon because that’s where the sellers are. Creating an Amazon clone is not impossible.
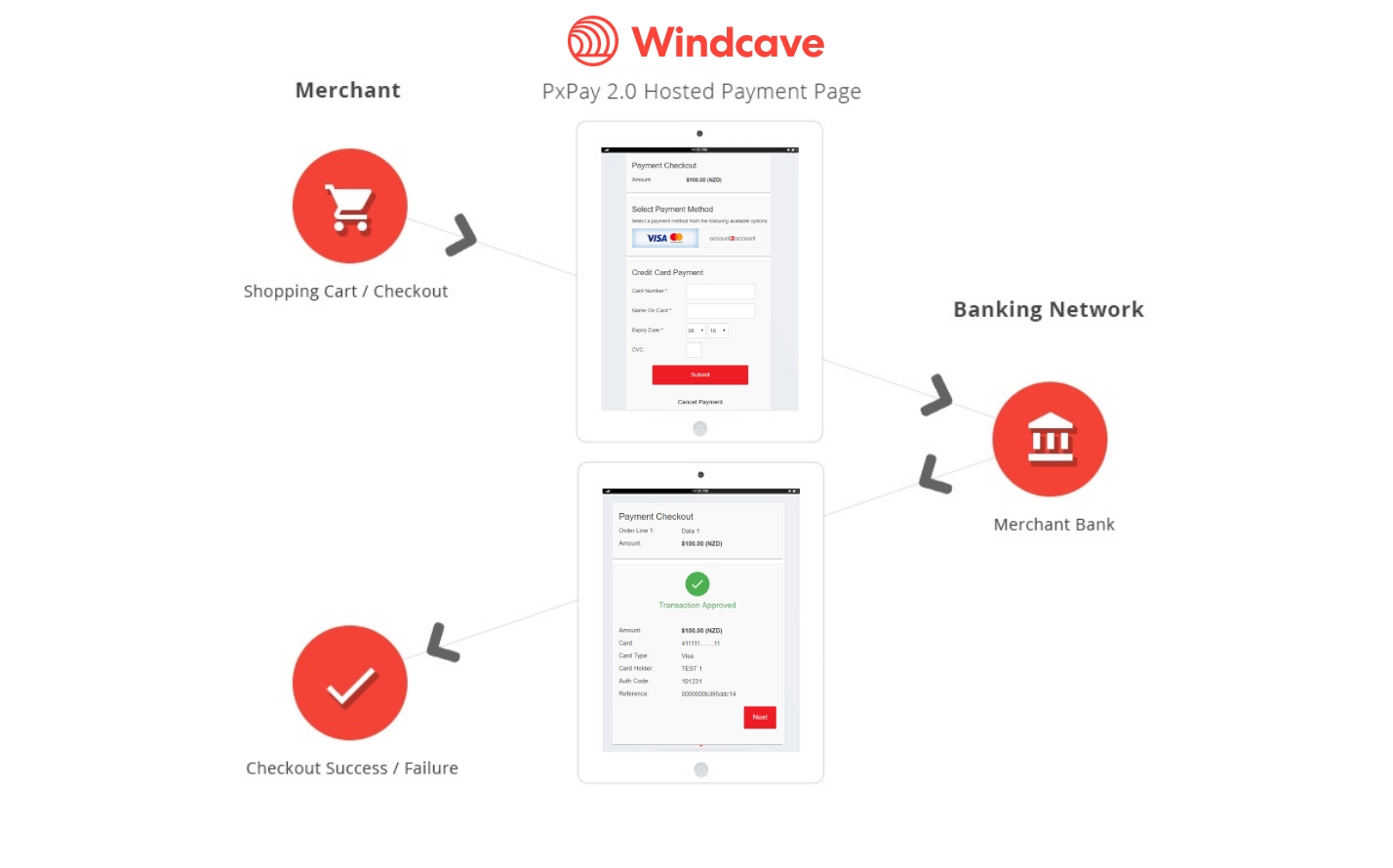
One benefit of delivering GPT-3 as a cloud service is that it removes the technical and financial challenges of running the AI model. And part of it will come from renting its huge language model to other companies. It needed a sustainable source of income. And it couldn’t continue operating on donations from founders and backers. The AI research lab is burning a lot of cash to train its AI models and cover the salaries of its scientists. OpenAI’s decision to commercialize GPT-3 was largely due to the company’s need for sustainable funding. And this would eventually lead to a new generation of entrepreneurs who would create new businesses on top of GPT-3.īut that’s not how the business of artificial intelligence works. This performance led to speculation that GPT-3 would enable developers to create AI-powered apps without extensive knowledge of deep learning. And for many applications, you just need to show the AI model one or two examples of the output you expect, and it starts to perform the task on new input with remarkable accuracy. This essentially means that you can use GPT-3 for many applications without writing any code, without spending time and expensive resources retraining it, and without making any tweaks to the architecture. The result was a language model that could perform zero-shot and few-shot learning.

GPT-3 surpassed its predecessor in size by more than two orders of magnitude and was trained on at least 10 times more data. But it is remarkable nonetheless and shows that you can still move the needle on NLP by creating even larger neural networks and feeding them more data than before.
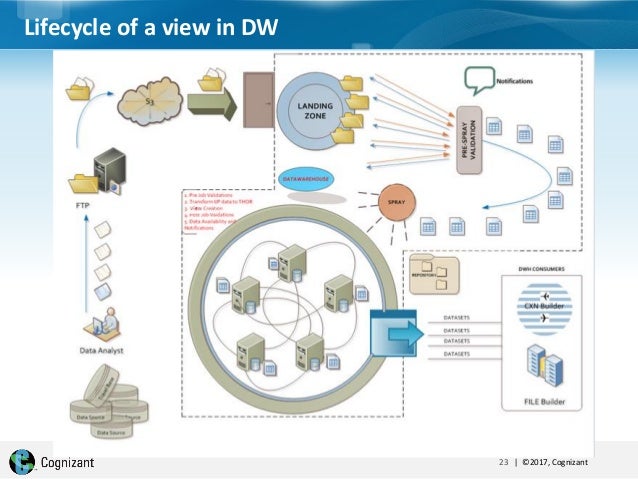
Like other language models that are based purely on deep learning, it struggles with common sense and isn’t good at dealing with abstract knowledge. GPT-3 from a scientific standpointĪs far as research in natural language processing is concerned, GPT-3 is not a breakthrough. But developments so far show that those who stand to benefit the most from GPT-3 are companies that already wield much of the power in AI, not the ones who want to start from scratch. Granted, a disruptive technology might need more time to create a sustainable market, and GPT-3 is unprecedented in many respects.
